From sending cancer into remission to alleviating Covid-19 symptoms, immunotherapy can provide revolutionary disease treatments. Immunotherapies use antibodies — proteins that bind to cell markers called antigens — to target and eliminate the antigen. But despite how effective immunotherapy can be, it isn’t widely used because finding the right antibodies to develop treatments is challenging, time-consuming work.
Georgia Tech researchers are making this process a little easier, though. Their new tool, AF2Complex, used deep learning to predict which antibodies could bind to Covid-19’s infamous spike protein. The researchers created input data for the deep-learning model using sequences of known antigen binders. This method correctly predicted 90% of the best antibodies in one test with 1,000 antibodies and was recently published in Proceedings of the National Academy of Sciences. Treating Covid-19 is just the start of its potential.
“AF2Complex improves therapeutic development,” said Mu Gao, a senior research scientist in the School of Biological Sciences (SBS). “If you have a high-quality model, then you can tinker with the protein sequence and optimize the antibody, making it more suitable for drug development.”
Developing the Deep-Learning Model
The researchers weren’t the first to use deep learning to predict protein structures, but they did considerably expand the model’s capabilities. In 2020, the Nobel Prize-winning DeepMind AlphaFold, an Alphabet project, made breakthroughs using deep learning to predict the protein structures of single proteins. Georgia Tech researchers pushed the model to predict the structures of protein complexes. In 2021, they created the first version of AF2Complex, which could predict interactions between multiple, complex proteins like E. coli. Applying it to human proteins was the next step — but much harder.
“Normally, when you predict protein-protein interactions, the surface area of the protein is quite large, so you could afford to make a few mistakes with an imperfect model,” said Jeffrey Skolnick, a Regents’ Professor and the Mary and Maisie Gibson Chair in SBS and a Georgia Research Alliance Eminent Scholar. “But an antibody-protein interaction occupies a much smaller interfacial area. Imagine going from hitting a big target anywhere to hitting the bullseye.”
Determining how to predict the antibody-antigen interactions was the biggest challenge. The researchers focused on the Covid-19 virus because it had many complex antigen-binding sequences and epitopes, the specific molecule region that interacts with B- and T-cells to trigger an immune response. Covid-19 also was a widely available dataset, unlike many immunotherapies to which only pharmaceutical companies have access. The Covid-19 database, in effect, offered a rich training environment for the AF2 algorithm.
Skolnick and Gao used Covid-19 sequences from known antibodies to identify evolutionary relationships and patterns, improving the accuracy of predictions. From there, they applied the AF2 deep-learning model, already trained on a vast amount of protein structure data. The model used sequences to predict how proteins fold and interact, developing a 3D structure of protein complexes. Plus, it could produce 3D structures for more than just one dominant epitope.
The predictions were validated against experimental data, refining the model. With these predicted structures, researchers can do everything from better understanding biological processes to developing new drugs.
Treating the Virus of the Future
The researchers believe deep-learning technologies could revolutionize how we treat future diseases. With infinite resources and time, researchers could manually try every antibody-antigen combination, but no scientist has that. AF2Complex can narrow the focus and get to the treatment sooner.
“Imagine the virus from hell arises. You could design a series of antibodies using this algorithm, so it cuts down the time for vaccine development,” Skolnick said. “There are no substitutes for a real experiment, but AF2Complex can prioritize which experiments you should do, so you have more shots at the goal.”
The researchers are already collaborating with Emory University to conduct experiments that validate AF2Complex’s findings. They also are pursuing a path to commercialize the model. When the next pandemic starts, we will be better prepared.
The National Institutes of Health provided, and the Department of Energy and National Science Foundation supported, the main computing resources.
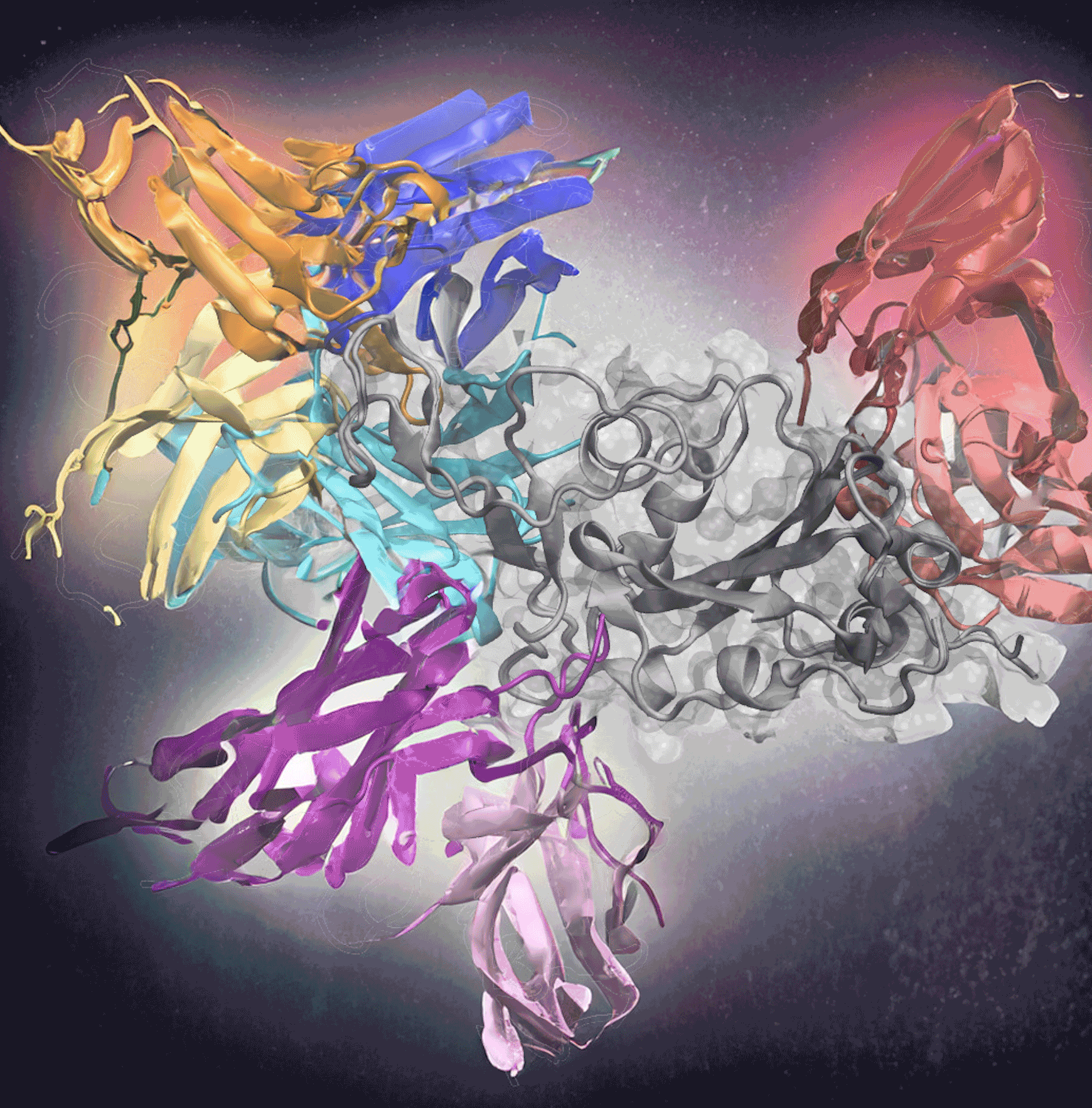
Deep learning effectively predicts antibodies targeting distinct epitopes on the SARS-CoV-2 spike protein (gray, center). [Image courtesy of Mu Gao; Illustration by Stephanie Stephens
Tess Malone, Senior Research Writer/Editortess.malone@gatech.edu