In partial fulfillment of the requirements for the degree of Doctor of Philosophy in Bioinformatics in the School of Biological Sciences Shashidhar Ravishankar Defended his thesis: Genetic epidemiology algorithms for tracking drug resistance variants and Genomic clustering of Plasmodium species Monday, August 12, 2019 10:00 AM Eastern Time Molecular Sciences and Engineering Building, (MoSE) Room 1201A Thesis Advisor: Dr. Fredrik O. Vannberg School of Biological Sciences Georgia Institute of Technology Committee members: Dr. King I. Jordan School of Biological Sciences Georgia Institute of Technology Dr. Eberhard O. Voit School of Biomedical Engineering Georgia Institute of Technology/Emory University Dr. John F. McDonald School of Biological Sciences Georgia Institute of Technology Dr. Venkatachalam Udhayakumar Malaria Branch Centers for Disease Control and Prevention Abstract: The goal of this thesis is to develop algorithms for the analysis of P. falciparum, P. brasilianum, and P. malariae. Malaria is endemic in many parts of the world including regions of central Africa, South America and South East Asia. Currently there are five known species that cause malaria in humans: P. falciparum, P. vivax, P. malariae, P. ovale and P. knowlesi. According to a World Health Organization (WHO) report from 2018, these five species were responsible for nearly 219 million infections, resulting in an estimated 435,000 deaths related to malaria in 2017. In this work, we highlight algorithms that can identify relatedness of the Plasmodium species and detect drug resistant P. falciparum parasites. The two specific aims in this proposal are designed towards improving the genetic epidemiology of Plasmodium species using novel algorithmic approaches. First, we describe a consensus based variant identification framework to identify SNPs with high confidence. We highlight its applicability in malaria surveillance by using the framework to track known markers associated with drug resistance in P. falciparum. Our results show that consensus based variant calling framework can be used to overcome the inherent bias of different variant calling algorithms as well as providing a metric to assess the confidence associated within any variant detected from NGS data. In the second aim, we describe a k-mer based alignment free algorithm to identify the relatedness of isolates from raw NGS data. Using a weighted Jaccard distance, we describe an exact method for estimation of distance between isolates from k-mer count data. The memory efficiency, scalability and accuracy of the algorithm was demonstrated using in-silico datasets generated from genomes of 12 Plasmodium species, as well as real-world isolates from an outbreak of C. auris in Colombia. The improved accuracy and scalability offered by the methods described in this work can have significant impact on the use of NGS towards understanding the genetic epidemiology of malaria and enable standardization of NGS analysis across public health laboratories.
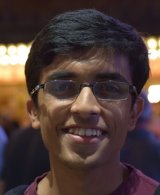
Shashidhar Ravishankar, Bioinformatics PhD