A holistic approach to improving ovarian cancer detection and management
In partial fulfillment of the requirements for the degree of
Doctor of Philosophy in Bioinformatics
in the School of Biological Sciences
Dongjo Ban
Defends his thesis:
A holistic approach to improving ovarian cancer detection and management
Friday, June 23rd, 2023
9:00 AM
Parker H. Petit Institute for Bioengineering and Biosciences, Suddath Seminar Room (IBB 1128)
Zoom Link = https://gatech.zoom.us/j/97641531005
Thesis Advisor:
Dr. John F. McDonald
School of Biological Sciences
Georgia Institute of Technology
Committee Members:
Dr. I. King Jordan
School of Biological Sciences
Georgia Institute of Technology
Dr. Jeffrey Skolnick
School of Biological Sciences
Georgia Institute of Technology
Dr. J. Todd Streelman
School of Biological Sciences
Georgia Institute of Technology
Dr. Mark Styczynski
School of Chemical and Biomolecular Engineering
Georgia Institute of Technology
Abstract:
Also known as the “silent killer”, ovarian cancer (OC) continues to pose a unique challenge due to its subtle early-stage symptoms and late diagnoses. To address this public health concern, this research aims to explore variations observed in OC, specifically at the genomic and metabolomic levels. By utilizing data generated from high-throughput technologies, potential strategies for early detection and improving treatment options are examined.
The first part of this study delves into the genomic aspect of OC, examining the tumor mutation burden (TMB) and patterns of copy-number alterations (CNAs). Higher TMB was found in localized tumors, with cancer-associated genes showing a higher TMB compared to non-cancer genes. Moreover, impaired DNA-repair mechanisms were observed to contribute to elevated TMB levels. Additionally, patterns of selective pressures, represented by 𝑑𝑁/𝑑𝑆 ratio estimates, differed between early- and late-stage OC. Concurrently, CNAs were analyzed to understand their impact on OC patients. A higher prevalence of amplification events compared to deletion events was observed, along with a higher number of affected genes in the early-stage group. Notably, CNAs were not found to be higher in cancer-associated genes relative to non-cancer genes, but examining the driver regions showed a preference for amplification in oncogenes and deletion in tumor suppressor genes. The second part of the study, focusing on metabolomics, utilized high-throughput serum metabolomic profiles from OC patients and non-cancerous individuals from different geographical locations with machine learning (ML) approaches. The classifiers demonstrated promising predictive potential, highlighting the utility of metabolomics in detecting early-stage OC. Furthermore, the emergence of lipid or lipid-like molecules as potential markers emphasizes their significance in OC detection.
Collectively, these findings highlight the feasibility of a multifaceted approach from which personalized cancer management strategies can be developed, considering the individual variations observed in patients. Consequently, high-risk individuals can be clinically identified for more frequent monitoring, and appropriate treatment options can be assigned to achieve optimal outcomes. Given the increasing amount of data and the continuous advancements in technology, comprehensive approaches have the capacity to enhance survival rates and improve the quality of life for OC patients.
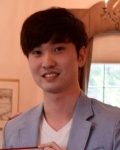