Analysis and design of multi-modal clinical and genomic risk scores for disease prediction using machine learning
In partial fulfillment of the requirements for the degree of
Doctor of Philosophy in Bioinformatics
in the School of Biological Sciences
Monica Isgut
Defends her thesis:
Analysis and design of multi-modal clinical and genomic risk scores for disease prediction using machine learning
Thursday, July 27th 2023
5:00 PM
Zoom Link = https://gatech.zoom.us/j/6872970574
Thesis Advisor:
Dr. May D. Wang
Department of Biomedical Engineering
Georgia Institute of Technology
Committee Members:
Dr. I. King Jordan
School of Biological Sciences
Georgia Institute of Technology
Dr. Yunan Luo
School of Computational Science and Engineering
Georgia Institute of Technology
Dr. Saurabh Sinha
Department of Biomedical Engineering
Georgia Institute of Technology
Dr. Blake Anderson
School of Medicine
Emory University
Abstract:
Polygenic risk scores (PRSs) are promising tools for leveraging genomic data for disease risk prediction in clinical settings. However, little is known about their value in the context of clinical data routinely available. This work aims to analyze the value-add of genomic data in multi-modality risk prediction models over models with clinical data alone, 1) for several diseases, 2) across disease subpopulation groups, and 3) across different categories of model complexity (i.e., logistic regression vs. neural networks) and clinical or genomic feature space.
The latter more specifically evaluates: a) the effect of integrating large-scale clinical data derived from electronic health records (EHRs) with PRSs in a multi-modal neural network on the estimated value-add of the PRSs in the risk model, and b) the effect of integrating standard small-scale clinical risk factors (i.e., body mass index, smoking status) with genomic data in the form of individual genomic features (hereafter also denoted as a PRS) in a neural network on the estimated value-add of the genomic data.
In addition to the systematic analysis of the factors contributing to the value-add of genomic data and the design of multi-modality genomic and clinical neural networks for disease prediction, this work also introduces two novel representation learning algorithms designed to derive low-dimensional representations of EHR diagnostic data and genotype data, respectively. Furthermore, this work explores various the use of neural network interpretability tools applied to multi-modality disease risk scores to gain insights into important or interacting features utilized in risk prediction.
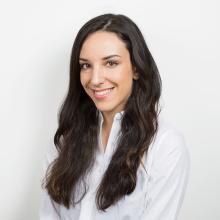