In partial fulfillment of the requirements for the degree of
Doctor of Philosophy in Bioinformatics
in the School of Biological Sciences
Tomáš Brůna
Defends his thesis:
Unsupervised Algorithms for Automated Gene Prediction in Novel Eukaryotic Genomes
Monday, July 18, 2022
2:00 PM Eastern Time
EBB Krone - Children's Healthcare of Atlanta Seminar Room (room #1005)
Zoom link: https://gatech.zoom.us/j/98875806845
Thesis Advisor:
Dr. Mark Borodovsky
School of Computational Science and Engineering and Department of Biomedical Engineering
Georgia Institute of Technology
Committee Members:
Dr. I. King Jordan
School of Biological Sciences
Georgia Institute of Technology
Dr. Jung H. Choi
School of Biological Sciences
Georgia Institute of Technology
Dr. Xiuwei Zhang
School of Computational Science and Engineering
Georgia Institute of Technology
Dr. Kostas T. Konstantinidis
School of Civil and Environmental Engineering
Georgia Institute of Technology
Summary:
Gene prediction, the identification of the location and structure of protein-coding genes in genomic sequences, is one of the first and most important steps in the analysis of assembled genomes. The exponential growth of sequenced eukaryotic genomes necessitates fully automated computational gene prediction methods. Due to the complexity and diversity of eukaryotic genomes, the task of accurate automatic eukaryotic gene prediction remains an open challenge. This work presents three novel gene prediction algorithms that address specific aspects of this challenge and thus improve over existing gene prediction methods.
The first part of this thesis describes GeneMark-EP+, an unsupervised gene prediction algorithm that uses homologous cross-species proteins to guide its model training and gene prediction steps. In contrast to existing homology-based gene finders, which can only extract information from proteins of closely related species, GeneMark-EP+ is designed to utilize proteins of any evolutionary distance, including remote homologs. Consequently, GeneMark-EP+ can fully exploit the information contained in large and ever-growing protein databases that are, unlike transcriptomic data, always readily available prior to a genome annotation project start. GeneMark-EP+ is shown to significantly improve over previous GeneMark versions, including ones integrating transcriptomic data.
In the second part, BRAKER2 is presented—a fully automated protein homology-based gene prediction pipeline that integrates GeneMark-EP+ with AUGUSTUS, an accurate gene finder that requires supervised training. By combining complementary strengths of these two gene prediction tools, BRAKER2 achieves state-of-the-art gene prediction accuracy in a fully unsupervised manner. The high gene prediction accuracy of BRAKER2 is demonstrated in tests on a wide range of plant and animal genomes. Further, it is shown that BRAKER2 compares favorably with MAKER2, one of the most popular gene prediction pipelines.
Finally, this thesis describes GeneMark-ETP+, a self-training gene prediction algorithm that simultaneously utilizes diverse information streams—genomic, transcriptomic, and protein homology—throughout all stages of its model training and gene prediction. This evidence integration is achieved by, among other things, creating a novel method for simultaneous gene prediction in transcripts and genomic DNA. Notably, GeneMark-ETP+ builds upon the previous work of this thesis: its training is fully unsupervised and proteins of any evolutionary distance are utilized. The integrative approach of GeneMark-ETP+ is demonstrated to reach better prediction accuracy compared with competing tools combining ab initio-, protein homology-, and transcriptome-based predictions.
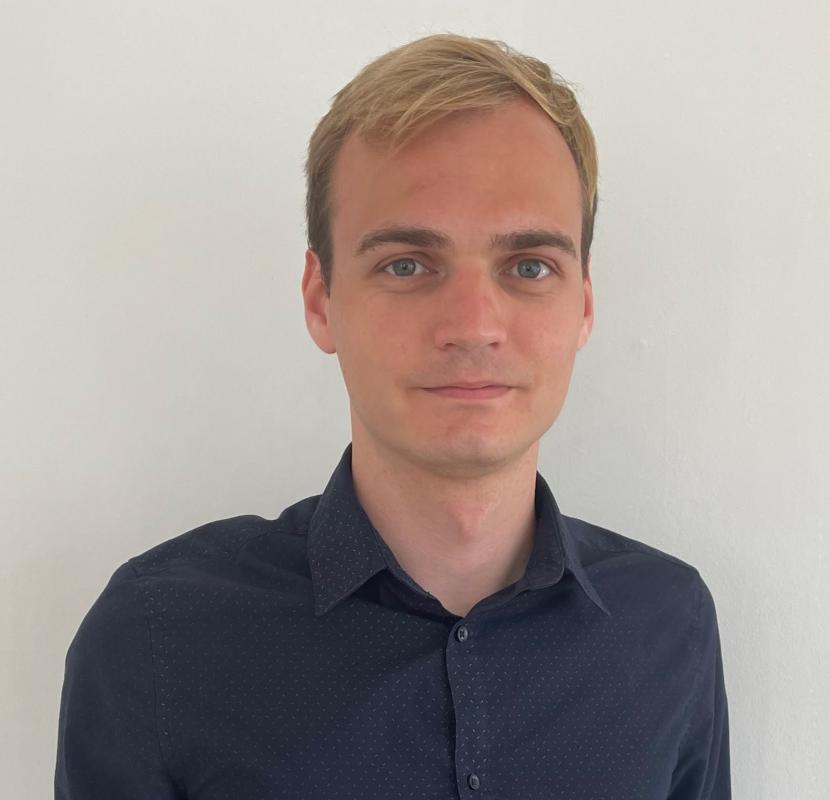